Displaying present location in the site.
ICT Global Trend Part7-4
Ethical AI Guidelines and Principle of Transparency (2/2)
This article is written by Mr.Yusuke Koizumi, Chief Fellow, Institute for International Socio-Economic Studies(NEC Group)
Ensuring transparency of AI in two ways
In the AI Ethics Guidelines of the European Commission’s HLEG explained in the previous report, “transparency” is listed as one of 7 key requirements. Transparency is extremely essential requirement in order that AI systems are widely accepted as indispensable in our society and are to gain trust and confidence among people.
One of the issues raised against machine-learning based AI is its feature as a black box. You may wish to know the reason why and how decision is made if you receive the unfavorable results of analysis that you are at high risk of heart disease after machine-learning based AI has analyzed your personal medical data. It is, however, acknowledged that machine-learning based AI is not capable of giving proper explanation in understandable language on how the decision has been made and what kind of logic and reasoning are behind there because machine-learning based AI is only to bring results based on statistical patters in the past.
As explained above it is a big issue that transparency of data analysis is properly ensured. There are two ways of ensuring transparency when companies are to develop and utilize AI. These are (1) transparency for consumers and (2) transparency for experts. (1) Transparency for consumers means that companies are pursuing explainability and are trying to design and manage the explanation easier for consumers to understand. (2) Transparency for experts means that companies are pursuing the auditability and accountability so that third-party experts can assure and verify the legitimacy of AI.
In(1)transparency for consumers, it is considered irrelevant that AI codes and technical details (All the parameters or their weight) are disclosed, but it is considered more relevant that explainability is secured by providing to consumers rational and reasonable explanation for them to understand without difficulty in response to their enquiry why such decision has been made by AI. This explainability consists of two steps. Namely the first step is to make publication of most popular explanation on decision-making process of AI such as main data impacting the judgement and their data sources. The second step is to make individual explanation in response to specific enquiry from consumers on some decision (in particular disadvantageous or unfavorable for them)
As one of the measures to explain how and why the decision is made, there is the explanation based on counterfactual assumption. This is to explain “you could obtain better decision if your data provided were satisfying certain level or conditions of decision-making criteria. For instance, when you apply for housing loan, but your application is rejected, you would be explained how much you have to earn annually to get your application approved. Explainable AI (XAI) is the challenge to support and enhance explainability in whole.
In (2) transparency for experts, not consumers but AI experts are involved to ensure the transparency. In USA there are companies who audit transparency, fairness and accuracy of AI and make voluntary certification. There is also a concept of “qualified transparency” where the proper understanding of relevant data protection authorities suffices even if consumers are not in full understanding of auditing report.
Privacy Impact Assessment (PIA) is the measure to identify negative impact on the protection of personal data at the system designing stage and avoid or mitigate the consequential impact. This measure is expected to reduce unexpected bias in particular. The preserving of input/output data is to contribute to the auditing and verification of error and to prevent similar errors in the future.
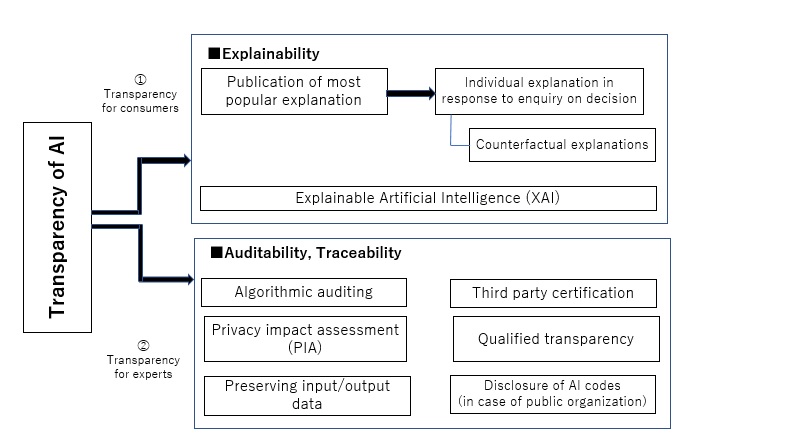